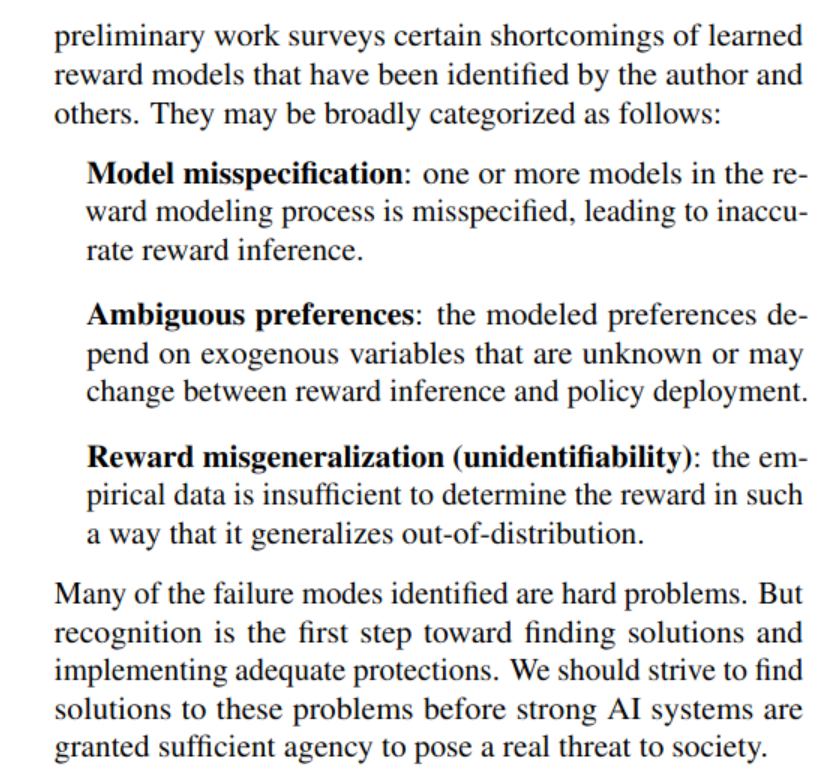
Silviu Pitis Failure Modes of Learning Reward Models for Sequence Model 2023
[TOC] Title: Failure Modes of Learning Reward Models for LLMs and other Sequence Models Author: Silviu Pitis Publish Year: ICML workshop 2023 Review Date: Fri, May 10, 2024 url: https://openreview.net/forum?id=NjOoxFRZA4¬eId=niZsZfTPPt Summary of paper C3. Preference cannot represented as numbers M1. rationality level of human preference 3.2, if the condition/context changes, the preference may change rapidly, and this cannot reflect on the reward machine A2. Preference should be expressed with respect to state-policy pairs, rather than just outcomes A state-policy pair includes both the current state of the system and the strategy (policy) being employed. This approach avoids the complication of unresolved stochasticity (randomness that hasn’t yet been resolved), focusing instead on scenarios where the outcomes of policies are already known. Example with Texas Hold’em: The author uses an example from poker to illustrate these concepts. In the example, a player holding a weaker hand (72o) wins against a stronger hand (AA) after both commit to large bets pre-flop. Traditional reward modeling would prefer the successful trajectory of the weaker hand due to the positive outcome. However, a rational analysis (ignoring stochastic outcomes) would prefer the decision-making associated with the stronger hand (AA), even though it lost, as it’s typically the better strategy. ...