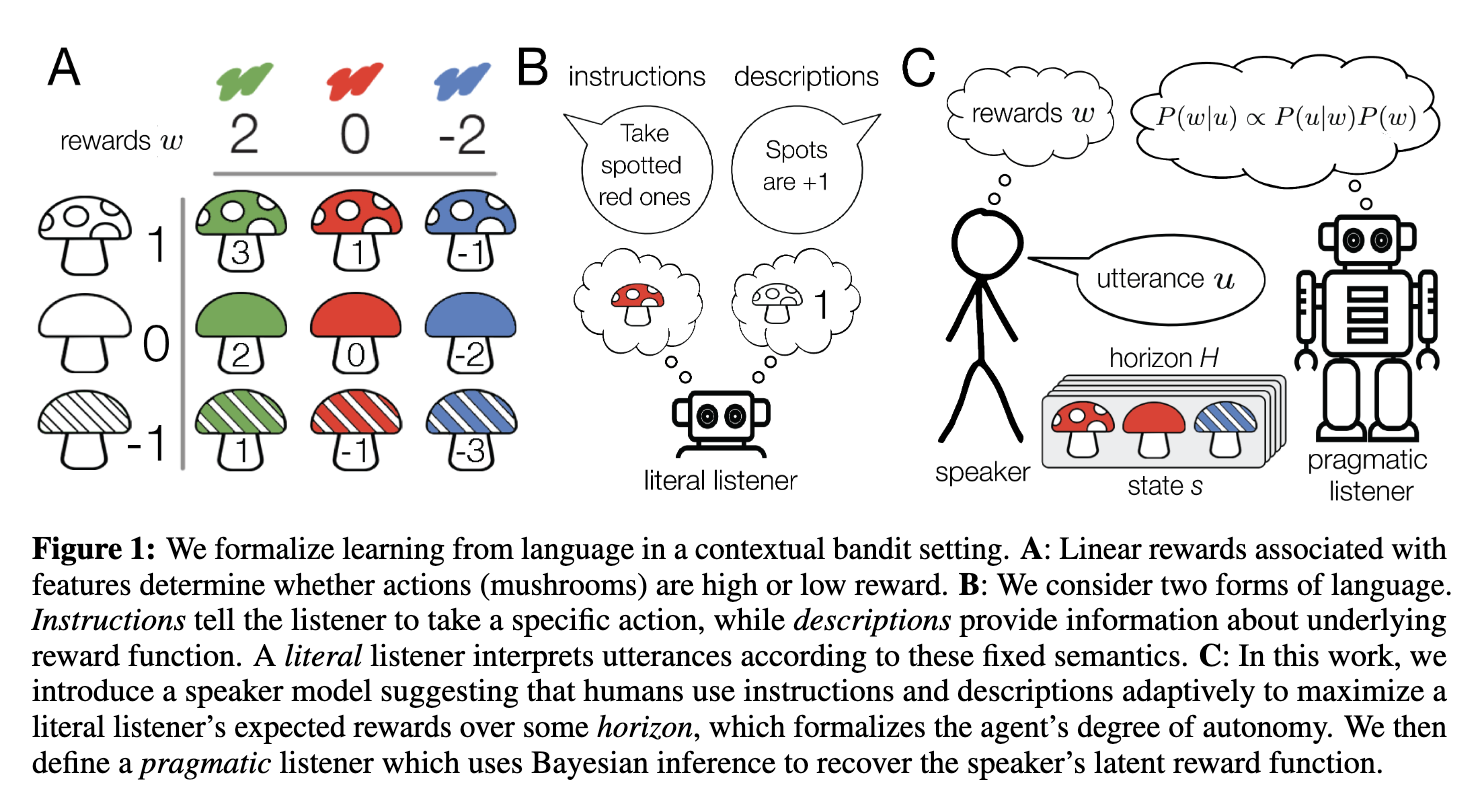
Theodore_r_sumers How to Talk So Ai Will Learn 2022
[TOC] Title: How to talk so AI will learn: Instructions, descriptions, and autonomy Author: Theodore R. Sumers et. al. Publish Year: NeurIPS 2022 Review Date: Wed, Mar 15, 2023 url: https://arxiv.org/pdf/2206.07870.pdf Summary of paper Motivation yet today, we lack computational models explaining such language use Contribution To address this challenge, we formalise learning from language in a contextual bandit setting and ask how a human might communicate preferences over behaviours. (obtain intent (preference) from the presentation (behaviour)) we show that instructions are better in low-autonomy settings, but descriptions are better when the agent will need to act independently. We then define a pragmatic listener agent that robustly infers the speaker’s reward function by reasoning how the speaker expresses themselves. (language reward module?) we hope these insights facilitate a shift from developing agents that obey language to agents that learn from it. Some key terms two distinct types of language ...