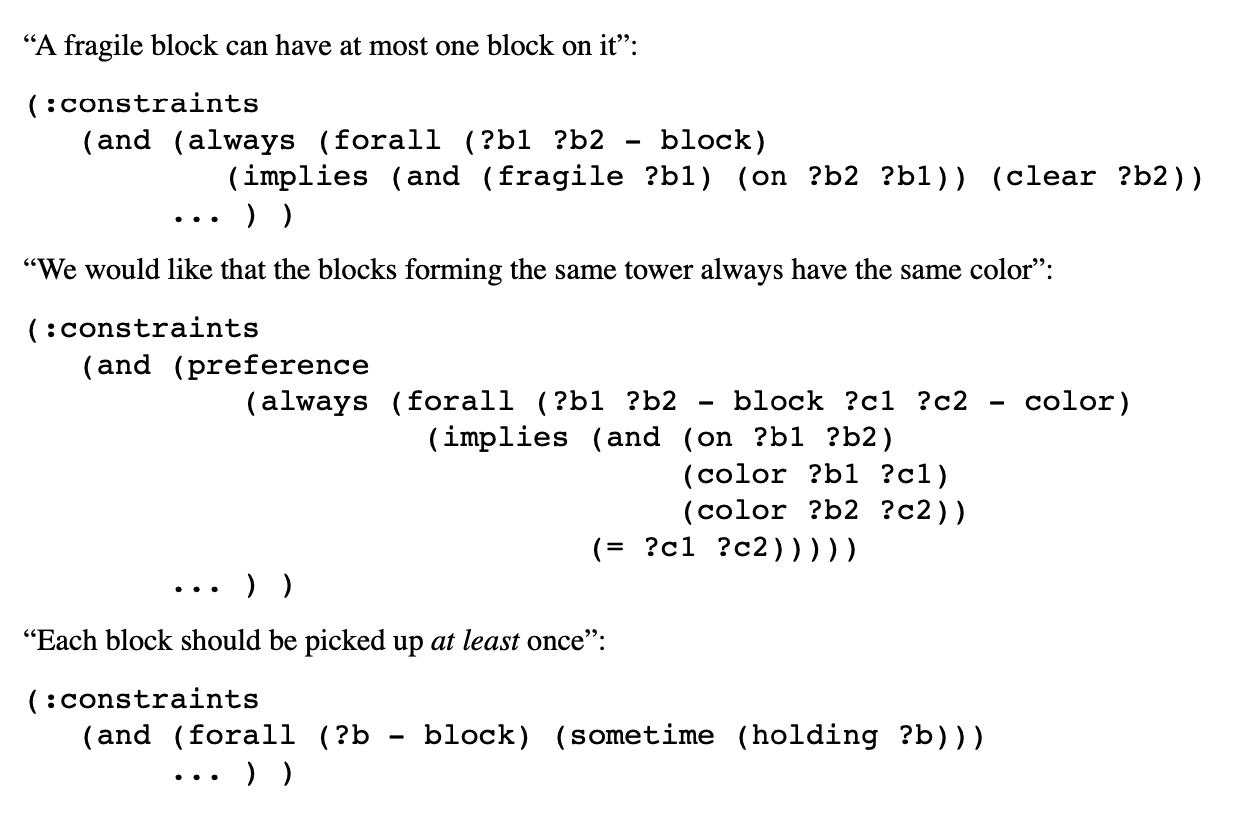
Gerevini Plan Constraints and Preferences in Pddl3 2005
[TOC] Title: Gerevini Plan Constraints and Preferences in PDDL3 Author: Alfonso Gerevini, Derek Long Publish Year: 2005 Review Date: Thu, Jan 11, 2024 url: http://www.cs.yale.edu/~dvm/papers/pddl-ipc5.pdf Summary of paper Motivation the notion of plan quality in automated planning is a practically very important issue. it is important to generate plans of good or optimal quality and we need to express the plan quality the proposed extended language allows us to express strong and soft constraints on plan trajectories i.e., constraints over possible actions in the plan and intermediate states reached by the plan as well as strong and soft problem goals. Some key terms some scenarios ...