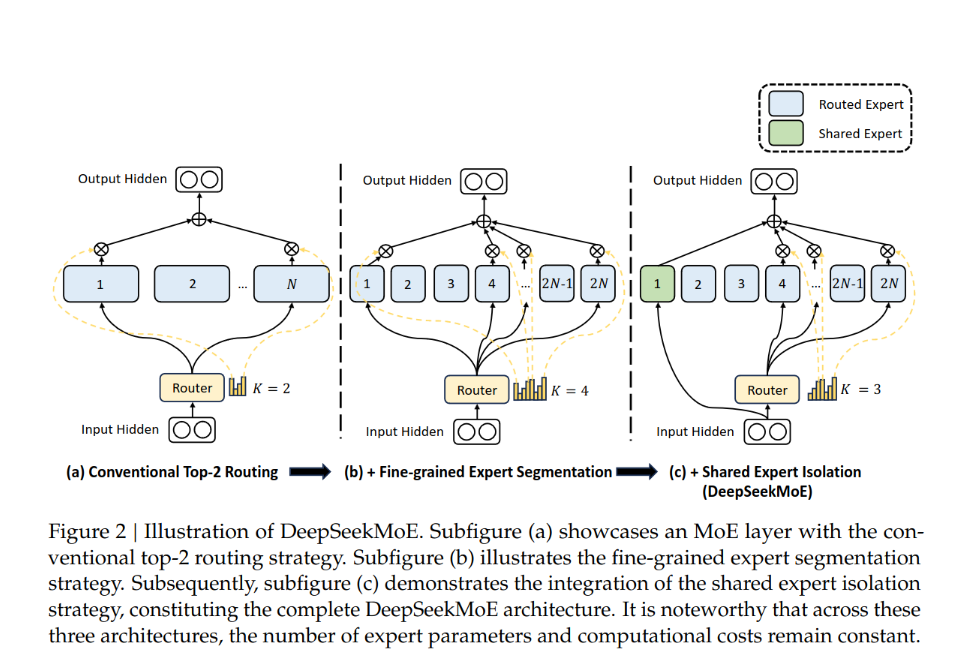
Damai Dai Deepseekmoe 2024
[TOC] Title: DeepSeekMoE: Towards Ultimate Expert Specialization in Mixture of Experts Language Models Author: Damai Dai et. al. Publish Year: 11 Jan 2024 Review Date: Sat, Jun 22, 2024 url: https://arxiv.org/pdf/2401.06066 Summary of paper Motivation conventional MoE architecture like GShard, which avtivate top-k out of N experts, face challenges in ensuring expert specialization, i.e., each expert acquires non-overlapping and focused knowledge, in response, we propose DeepSeekMoE architecture towards ultimate expert specialization Contribution segmenting expert into mN ones and activating mK from them isolating K_s, experts as shared ones, aiming at capturing common knowledge and mitigating redundancy in routed experts Some key terms MoE architecture...