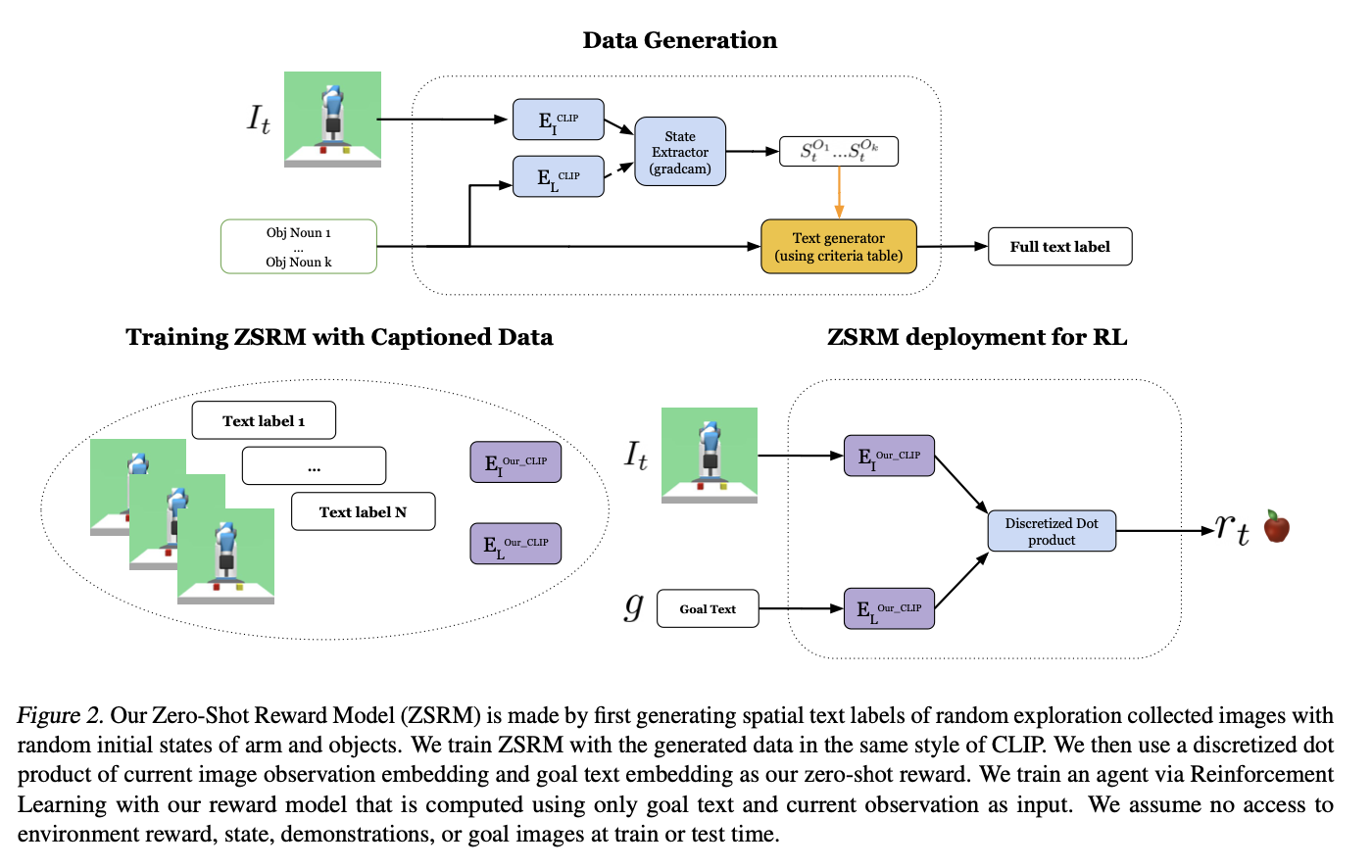
Parsa Mahmoudieh Zero Shot Reward Specification via Grounded Natural Language 2022
[TOC] Title: Zero Shot Reward Specification via Grounded Natural Language Author: Parsa Mahnoudieh et. al. Publish Year: PMLR 2022 Review Date: Sun, Jan 28, 2024 url: Summary of paper Motivation reward signals in RL are expensive to design and often require access to the true state. common alternatives are usually demonstrations or goal images which can be label intensive on the other hand, text descriptions provide a general low-effect way of communicating. previous work rely on true state or labelled expert demonstration match, this work directly use CLIP to convert the observation to semantic embeddings Contribution Some key terms Difference ...