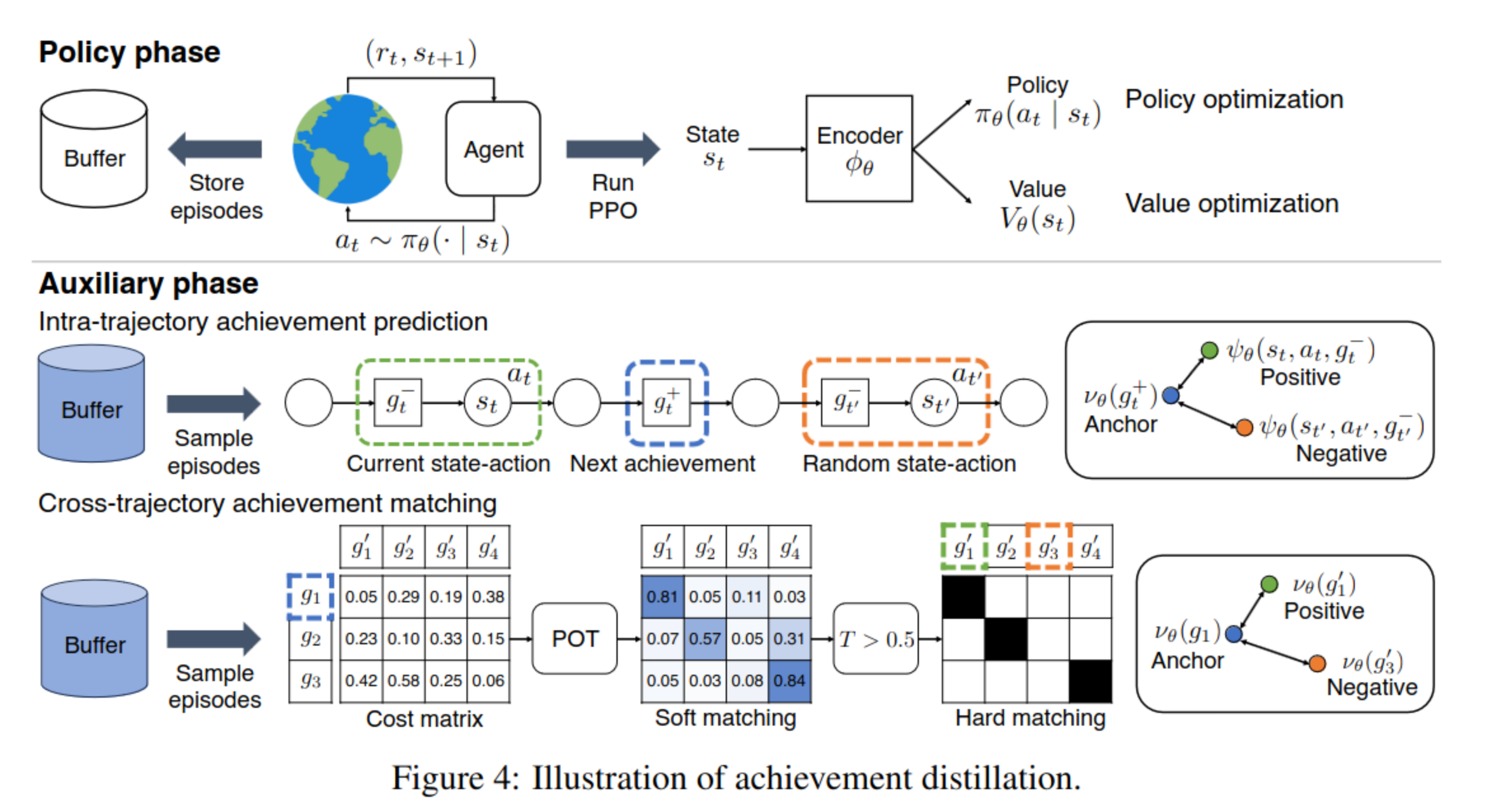
Discover Hierarchical Achieve in Rl via Cl 2023
[TOC] Title: Discovering Hierarchical Achievements in Reinforcement Learning via Contrastive Learning Author: Seungyong Moon et. al. Publish Year: 2 Nov 2023 Review Date: Tue, Apr 2, 2024 url: https://arxiv.org/abs/2307.03486 Summary of paper Contribution PPO agents demonstrate some ability to predict future achievements. Leveraging this observation, a novel contrastive learning method called achievement distillation is introduced, enhancing the agent’s predictive abilities. This approach excels at discovering hierarchical achievements, Some key terms Model based and explicit module in previous studies are not that good...