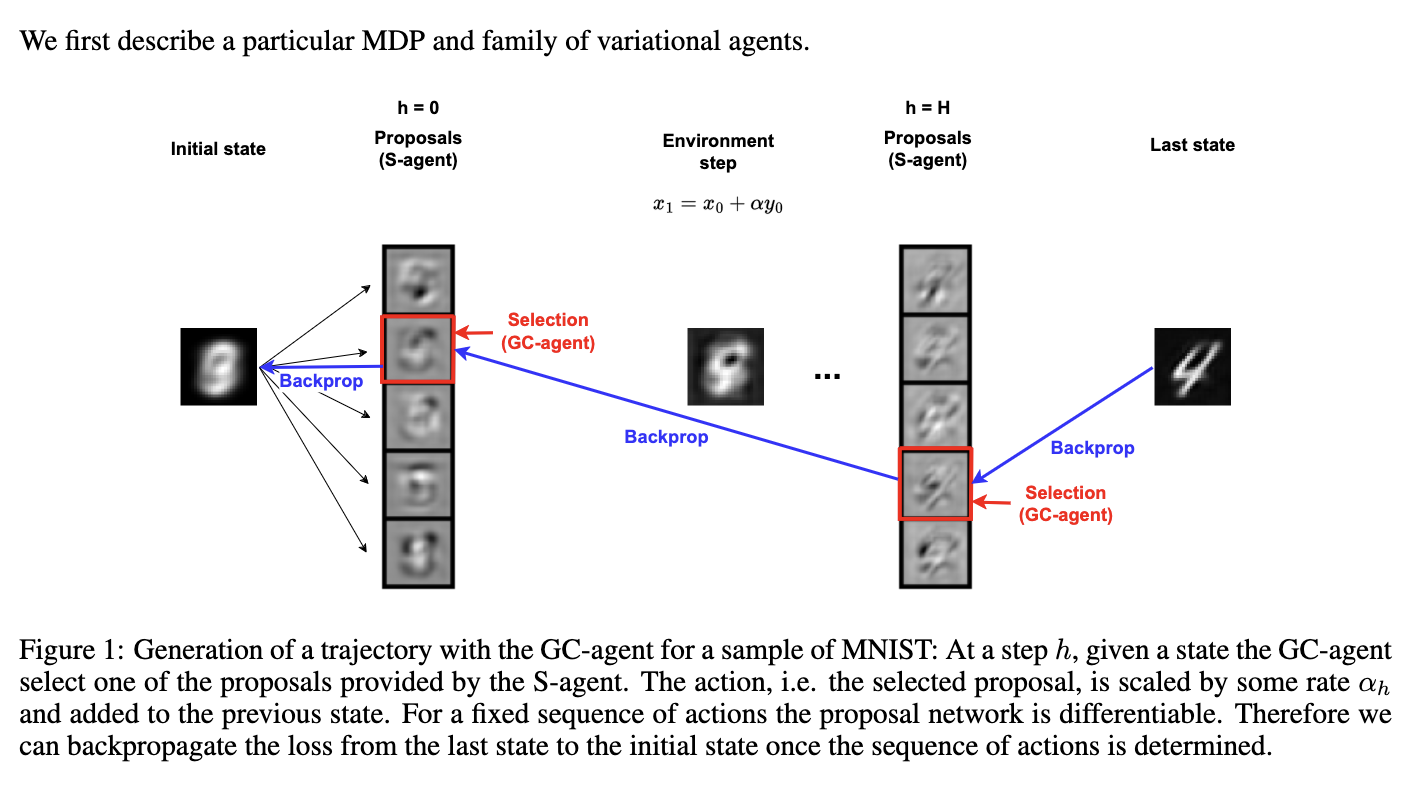
Mariana_learning Generative Models With Goal Conditioned Reinforcement Learning 2023
[TOC] Title: Learning Generative Models With Goal Conditioned Reinforcement Learning Author: Mariana Vargas Vieyra et. al. Publish Year: 26 Mar 2023 Review Date: Thu, Mar 30, 2023 url: https://arxiv.org/abs/2303.14811 Summary of paper Contribution we present a novel framework for learning generative models with goal-conditioned reinforcement learning we define two agents, a goal conditioned agent (GC-agent) and a supervised agent (S-agent) Given a user-input initial state, the GC-agent learns to reconstruct the training set. In this context, elements in the training set are the goals. during training, the S-agent learns to imitate the GC-agent while remaining agnostic of the goals At inference we generate new samples with S-agent. Some key terms Goal-Conditioned Reinforcement Learning (GCRL) framework ...