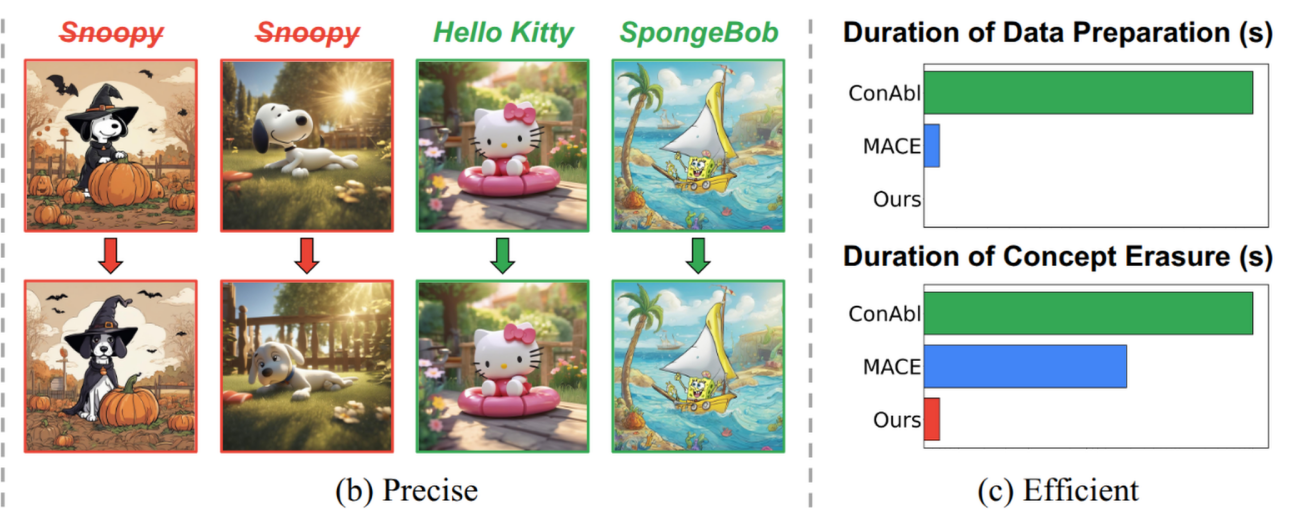
SPEED: Scalable, Precise, and Efficient Concept Erasure for Diffusion Models
[TOC] Title: SPEED: Scalable, Precise, and Efficient Concept Erasure for Diffusion Models Author: Ouxiang Li, Xinting Hu et. al. Publish Year: Mar 2025 Review Date: Wed, Apr 2, 2025 url: https://arxiv.org/abs/2503.07392 1 # input bibtex here Prerequisite: Training Networks in Null Space of Feature Covariance for Continual Learning https://arxiv.org/abs/2103.07113 Prerequisite knowledge projection on to a subspace of a vector Suppose $ U = {\mathbf{u}_1, \mathbf{u}_2, \ldots, \mathbf{u}_k} $ are the orthogonal vectors spanning the subspace $ S $. Construct a matrix $ \mathbf{U} $ whose columns are these vectors: $$ \mathbf{U} = [\mathbf{u}_1 , \mathbf{u}_2 , \cdots , \mathbf{u}_k] $$ Here, $ \mathbf{U} $ is an $ n \times k $ matrix, where $ n $ is the dimension of the ambient space (e.g., $ \mathbb{R}^n $), and $ k $ is the number of basis vectors (the dimension of $ S $). ...