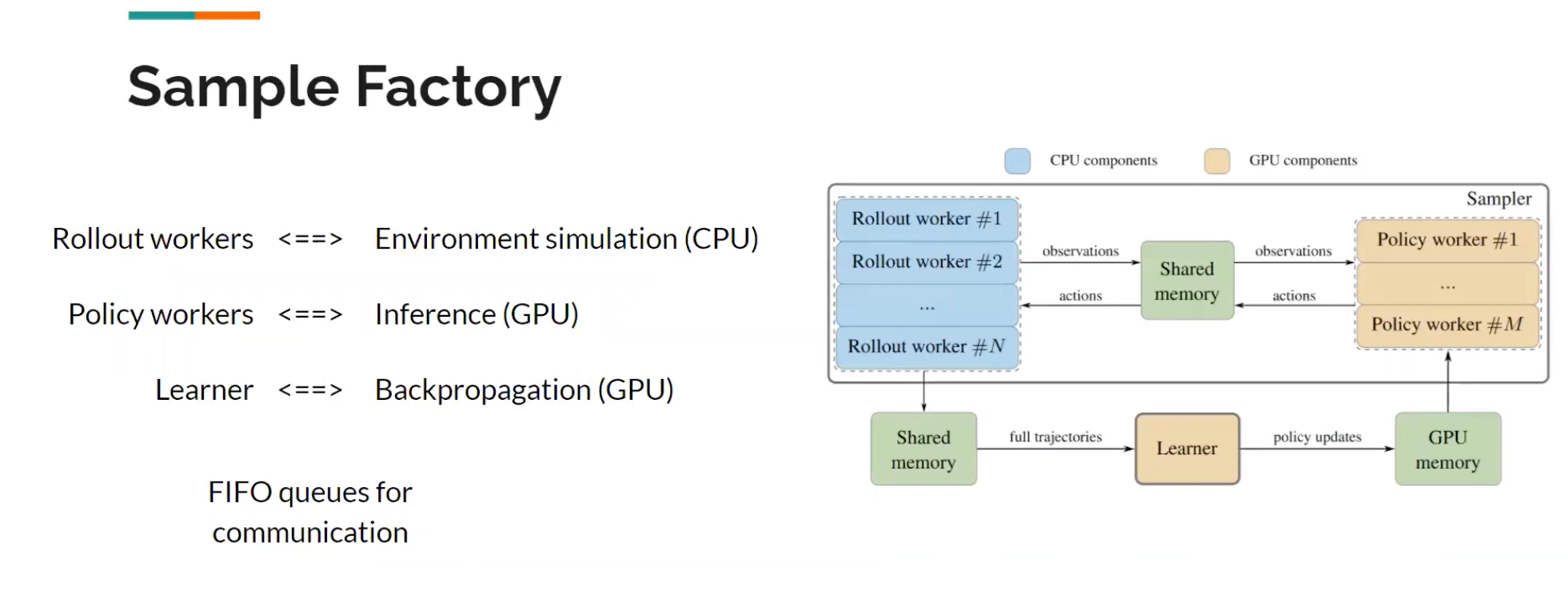
Alex_petrekno Sample Factory Asynchronous Rl at Very High Fps 2020
[TOC] Title: Sample Factory: Asynchronous Rl at Very High FPS Author: Alex Petrenko Publish Year: Oct, 2020 Review Date: Sun, Sep 25, 2022 Summary of paper Motivation Identifying performance bottlenecks RL involves three workloads: environment simulation inference backpropagation overall performance depends on the lowest workload In existing methods (A2C/PPO/IMPALA) the computational workloads are dependent -> under-utilisation of the system resources. Existing high-throughput methods focus on distributed training, therefore introducing a lot of overhead such as networking serialisation, etc. ...