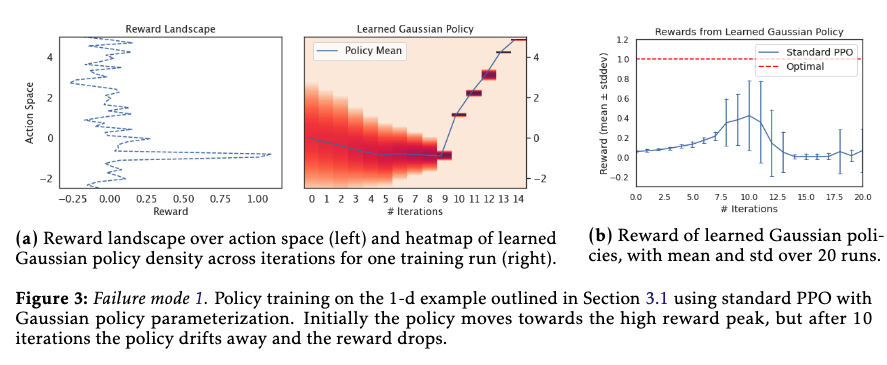
Chloe_ching_yun_hsu Revisiting Design Choices in Proximal Policy Optimisation 2020
[TOC] Title: Revisiting Design Choices in Proximal Policy Optimisation Author: Chloe Ching-Yun Hsu et. al. Publish Year: 23 Sep 2020 Review Date: Wed, Dec 28, 2022 Summary of paper Motivation Contribution on discrete action space with sparse high rewards, standard PPO often gets stuck at suboptimal actions. Why analyze the reason fort these failure modes and explain why they are not exposed by standard benchmarks In summary, our study suggests that Beta policy parameterization and KL-regularized objectives should be reconsidered for PPO, especially when alternatives improves PPO in all settings. The author proved the convergence guarantee for PPO-KL penalty version, as it inherits convergence guarantees of mirror descent for policy families that are closed under mixture Some key terms design choices ...