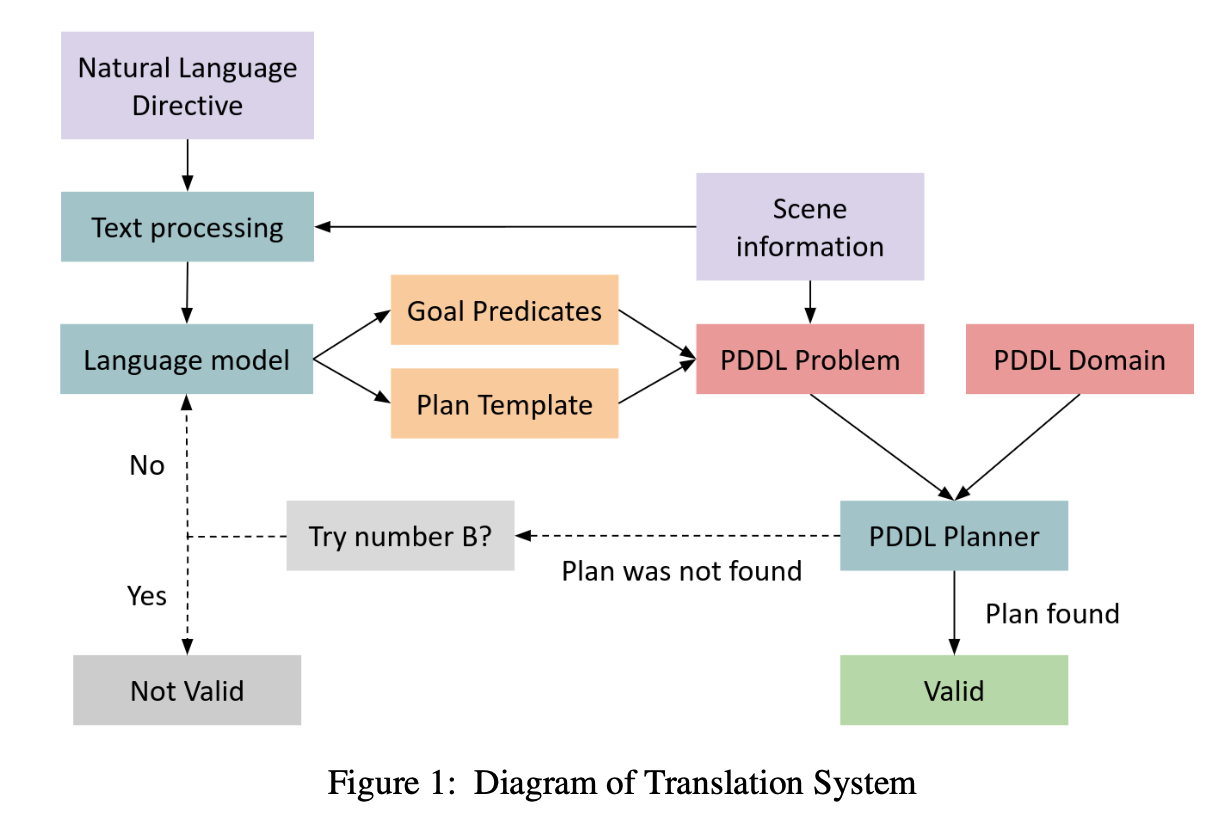
Avichai Levy Understanding Natural Language in Context 2023
[TOC] Title: Understanding Natural Language in Context Author: Avichai Levy et. al. Publish Year: ICAPS 2023 Review Date: Mon, Jan 29, 2024 url: https://ojs.aaai.org/index.php/ICAPS/article/view/27248 Summary of paper Contribution The paper discusses the increasing prevalence of applications with natural language interfaces, such as chatbots and personal assistants like Alexa, Google Assistant, Siri, and Cortana. While current dialogue systems mainly involve static robots, the challenge intensifies with cognitive robots capable of movement and object manipulation in home environments. The focus is on cognitive robots equipped with knowledge-based models of the world, enabling reasoning and planning. The paper proposes an approach to translate natural language directives into the robotโs formalism, leveraging state-of-the-art large language models, planning tools, and the robotโs knowledge of the world and its own model. This approach enhances the interpretation of directives in natural language, facilitating the completion of complex household tasks. ...