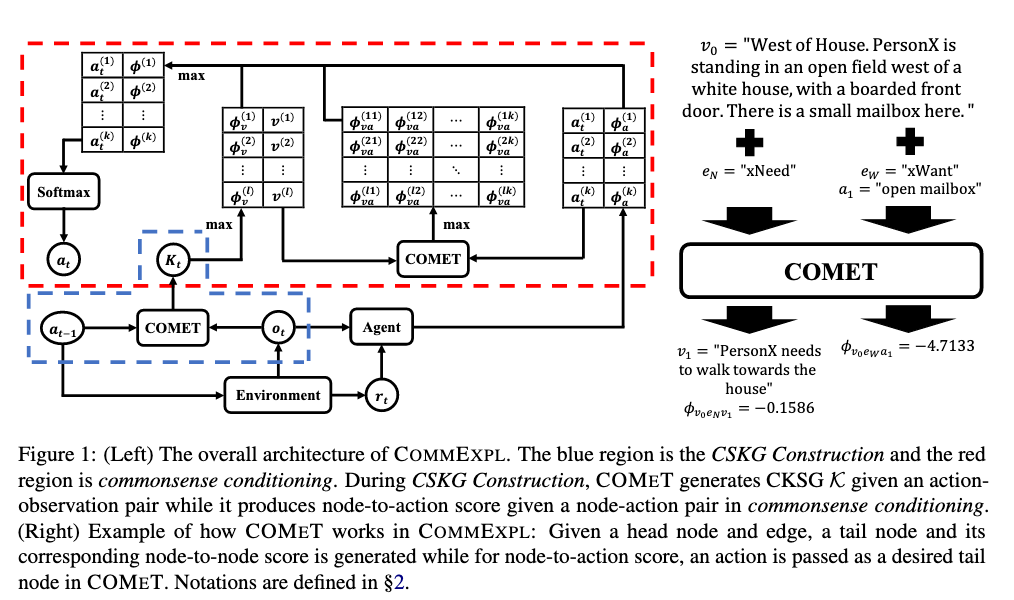
Dongwon Fire Burns Sword Cuts Commonsense Inductive Bias for Exploration in Text Based Games 2022
[TOC] Title: Fire Burns, Sword Cuts: Commonsense Inductive Bias for Exploration in Text Based Games Author: Dongwon Kelvin Ryu et. al. Publish Year: ACL 2022 Review Date: Thu, Sep 22, 2022 Summary of paper Motivation Text-based games (TGs) are exciting testbeds for developing deep reinforcement learning techniques due to their partially observed environments and large action space. A fundamental challenges in TGs is the efficient exploration of the large action space when the agent has not yet acquired enough knowledge about the environment. So, we want to inject external commonsense knowledge into the agent during training when the agent is most uncertain about its next action. Contribution In addition to performance increase, the produced trajectory of actions exhibit lower perplexity, when tested with a pre-trained LM, indicating better closeness to human language. Some key terms Exploration efficiency ...