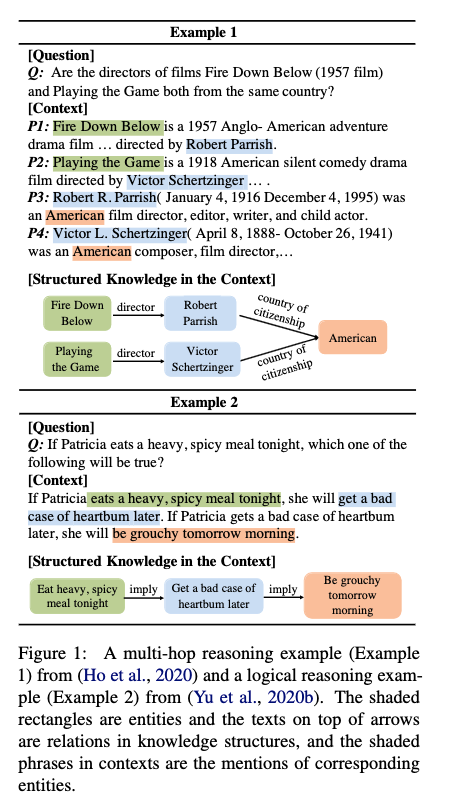
Siyuan_wang Unifying Structure Reasoning and Language Model Pre Training for Complex Reasoning 2023
[TOC] Title: Unifying Structure Reasoning and Language Model Pre Training for Complex Reasoning Author: Siyuan Wang et. al. Publish Year: 21 Jan 2023 Review Date: Wed, Feb 8, 2023 url: https://arxiv.org/pdf/2301.08913.pdf Summary of paper Motivation language models still suffer from a heterogeneous information alignment problem and a noisy knowledge injection problem. for complex reasoning, the context contains rich knowledge that typically exists in complex and sparse form. Contribution we propose to unify structure reasoning and language model pre-training identifies four types of elementary knowledge structures from contexts to construct structured queries utilise box embedding method to conduct explicit structure reasoning along query during language modeling Some key terms What is the problem ...